9 Ways to Use the Power of Exploratory Data Analysis for Marketing
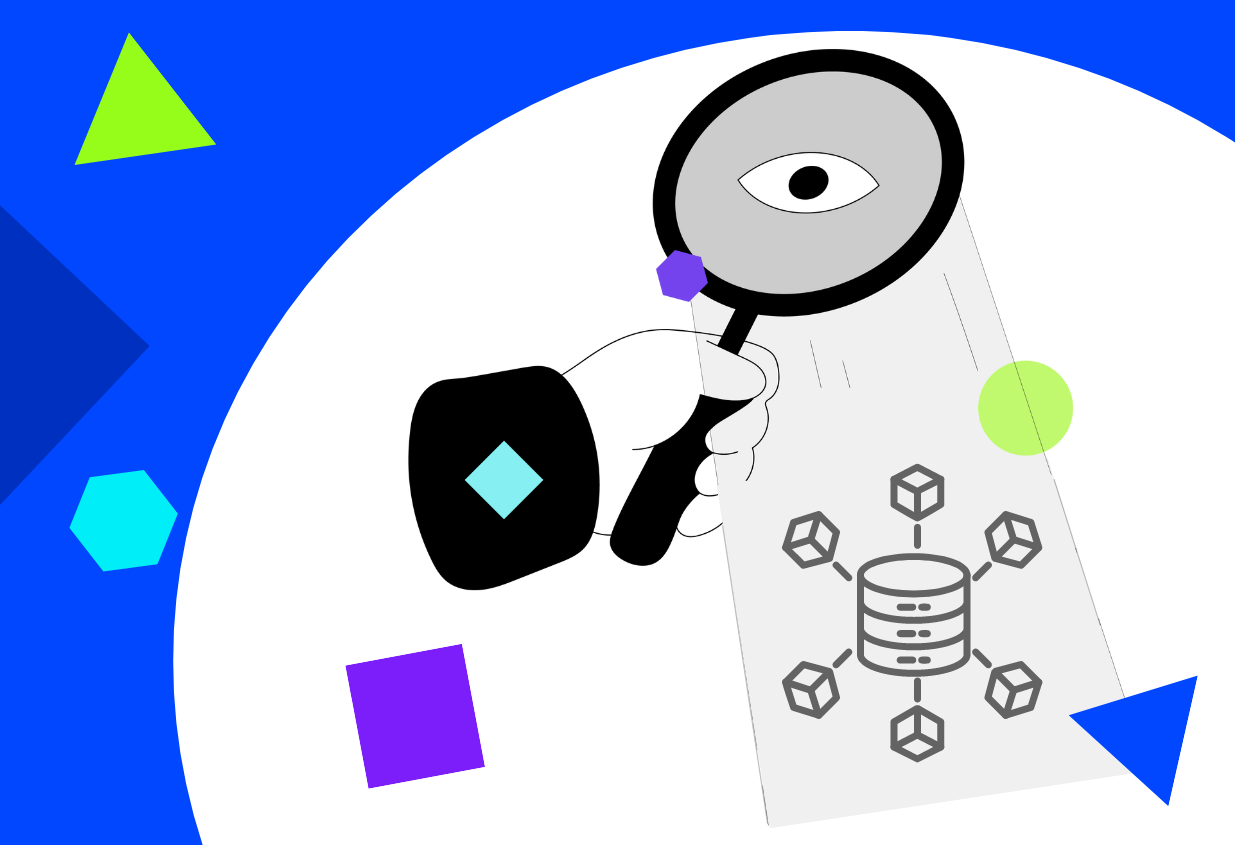
Anyone working in modern marketing knows that managing and interpreting vast amounts of data is a key factor in driving successful campaigns. For marketing agencies, effective marketing data management can make or break their ability to deliver meaningful insights and results for clients.
At the heart of every successful data analysis process is Exploratory Data Analysis (EDA)—a crucial step that ensures data is clean, reliable, and ready to fuel insightful marketing strategies.
Why EDA Is Essential for Marketing Data Analysis
EDA is not just a preliminary step in the data analysis workflow—it’s where analysts truly get to know the data, uncover patterns, and detect potential issues. By thoroughly understanding the data before diving into modeling or advanced analysis, you reduce the risk of inaccuracies, misinterpretations, and missed opportunities.
When done right, EDA sets the stage for successful marketing data analysis, ensuring that your reports and insights are not just accurate but truly data-driven. Here are nine key EDA tactics that will enhance your data analysis for marketing and help you deliver more precise and actionable insights.
1. Detect Outliers
Outliers can skew the results of your analysis and lead to misleading conclusions. Whether you're dealing with campaign performance data or customer demographics, it's essential to identify and handle outliers appropriately. Setting thresholds to detect outliers in real-time allows for quick action before they impact a campaign's performance.
2. Handle Missing Data
Missing data is one of the most common challenges in data analysis for marketing. Whether it’s due to platform discrepancies or incomplete records, missing data can throw off your entire analysis. As a data analyst, ensuring that all gaps are accounted for before diving into deeper analysis is crucial.
3. Check for Consistent Variable Types
Ensuring consistency in variable types across your datasets is a cornerstone of accurate marketing data analysis. When numeric values, dates, or text entries are misclassified, it can lead to errors in interpretation or modeling. This step is often overlooked but is critical in maintaining the quality and integrity of your analysis.
4. Examine Distinct Values in Categorical Data
In data analysis for marketing, categorical data—such as customer segments, campaign types, or ad formats—can be just as important as numerical data. It’s essential to verify that all distinct values in your dataset are accounted for and consistent across platforms.
5. Identify Anomalies in Campaign Data
Anomalies in marketing data often signal deeper issues—whether it’s a problem in the data collection process or an external factor affecting campaign performance. Anomaly detection is critical for keeping your data clean and your insights valid.
6. Understand Your Variables
Knowing your variables inside and out is a cornerstone of solid data management. This means checking for collinearity—when two or more variables are highly correlated—and understanding how they may influence each other. Exploring the relationships between different variables through easy-to-interpret graphs and tables enables more informed decision-making and helps avoid redundancy in marketing data models.
7. Visualize Key Marketing Metrics
Clear data visualization is an indispensable part of effective data analysis for marketing. Charts, graphs, and heatmaps help reveal trends, patterns, and insights that are not always obvious from raw data. Visualization tools enable faster, more intuitive understanding of complex datasets, making it easier to communicate key findings to stakeholders.
8. Understand Data Distributions
Analyzing the distribution of your marketing data is critical for selecting the right models and approaches. For example, if your data is not normally distributed, applying a model that assumes normal distribution could result in inaccurate predictions.
9. Understand Your Variables for Informed Decision-Making
In data analysis for marketing, knowing your variables inside and out is essential to producing accurate, actionable insights. This means checking for collinearity—when two or more variables are highly correlated—and understanding how they may influence each other. Collinearity can distort models and lead to misinterpretations, so it’s vital to identify and address it early in the analysis process.
The Power of EDA in Data-Driven Marketing
Exploratory Data Analysis is a critical step in managing and understanding marketing data. It’s not just about cleaning up the data, but about gaining a deeper understanding of it—what’s working, what’s not, and why.
By leveraging EDA, agencies and analysts can turn raw information into meaningful, actionable insights for marketing that drive smarter decisions and better campaign outcomes. Whether it's detecting outliers, managing missing data, or visualizing key metrics, adopting EDA best practices ensures that your analysis is accurate, reliable, and ready to guide strategic moves.
With NinjaCat, you have the tools to take full advantage of EDA, empowering your team to deliver data-driven results that matter. For agencies managing complex cross-channel campaigns or analysts diving deep into performance optimization, NinjaCat helps you transform data chaos into clarity, making every insight count.