How to Apply Marketing Data Science Principles for Smarter Data Management
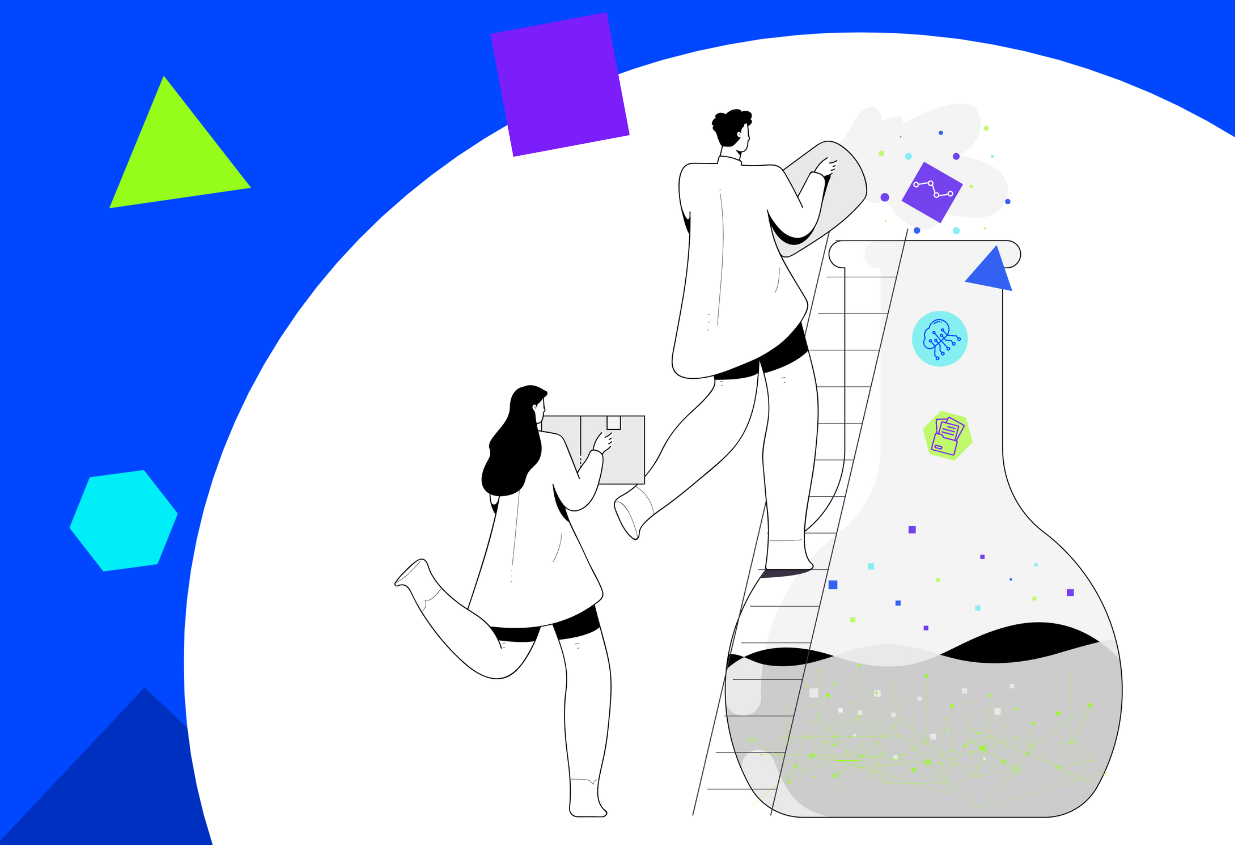
Marketing data management is increasingly challenging, given the volume and complexity of data at marketers' disposal. Enter marketing data science—a discipline that combines the best of data science with marketing data strategies to help you manage, analyze, and extract actionable insights from your data. By leveraging key principles of marketing data science, agencies can streamline data management processes and make more informed decisions.
Start with Simpler Models and Scale Up as Needed
In marketing data science, starting with simple models is a key principle. Applying this approach to marketing data management helps you focus on the metrics that truly matter, without getting overwhelmed by complex algorithms right away. By using straightforward analytics—like tracking Click-Through Rate (CTR), Cost Per Acquisition (CPA), conversion rates, or Return on Ad Spend (ROAS)—you lay the groundwork for more advanced insights down the road.
For many agencies, basic digital marketing dashboards that highlight these key performance indicators (KPIs) are a critical first step. These simple models offer a clear view of the campaign metrics that have the most direct impact. For instance, if you're running a social media campaign, start by evaluating how different types of content perform through engagement rates and conversions. This helps you understand your audience’s preferences without overcomplicating the data analysis.
Why this matters: Starting with simpler models not only helps build a solid foundation of understanding but also makes it easier to scale up to more advanced data science techniques as needed. Simple metrics can often provide the actionable insights necessary to refine your marketing strategies without the added complexity. As you advance, you'll have a deeper understanding of your data landscape, allowing you to make smarter decisions.
Example: Instead of jumping straight into complex predictive models for customer lifetime value, start by tracking more accessible metrics like customer acquisition costs and repeat purchase rates. Once these basic metrics are optimized, you can move to more sophisticated techniques, such as regression analysis or customer segmentation, if the initial data doesn’t fully address your strategic needs.
Master Exploratory Data Analysis (EDA): Understand Your Data First
In marketing data science, Exploratory Data Analysis (EDA) is a crucial first step. It helps marketers dig into their datasets to identify patterns, correlations, and outliers before applying advanced models. By understanding these data characteristics, you can ensure that your marketing data science strategies are built on a solid, informed foundation.
Use statistical techniques to analyze trends, spot correlations, and flag outliers. For example, check campaign performance data for seasonal spikes or drops. Segment your audience to see if particular demographics are more engaged during certain periods or with specific content. During this phase, it’s important to address any data inconsistencies that might distort your results—this could mean identifying duplicate records, missing data, or outliers that need investigation.
Why this matters: EDA helps you avoid misinterpretations and errors that can arise from incomplete or misleading data. By thoroughly exploring your data, you can uncover the trends and relationships that matter most—whether it’s seasonality in campaign performance or audience segments that overperform. For example, your client might see higher engagement in Q4 or notice different patterns within key demographics like age or location.
Example: Before attributing sales to specific marketing channels, conduct an EDA of customer journey data to identify which touchpoints are most frequently engaged. This will provide a clearer picture of customer behavior and guide the development of your attribution model.
Trust the Data, Not Your Bias
In marketing data science, it’s crucial to approach data with an open mind, free from personal bias. The same is true in marketing. Marketers often have preconceived notions about what works and what doesn’t, but letting those biases influence data interpretation can lead to missed opportunities and poor strategy.
Instead, let the data speak for itself. If you’re testing a new marketing campaign, analyze the data without any preconceived notions. Did the campaign drive the desired conversions, or did a different channel unexpectedly deliver better results? Always A/B test new strategies, and use statistical analysis to validate which changes lead to meaningful improvements. Your gut may tell you that a flashy new video ad should outperform a static image, but the data might reveal a different story.
Why this matters: Marketing trends change rapidly, and what worked last year might not work today. Trusting in the data rather than sticking to what "feels right" allows you to adapt your strategy in line with current market dynamics.
Example: During a campaign, you might believe that a new influencer partnership will be the key driver of sales. However, post-campaign analysis could show that a simple email marketing sequence actually generated more revenue. Use this insight to optimize future strategies, relying on the data rather than intuition.
Experiment and Iterate: Don’t Over-Tune One Model
In marketing data science, constant experimentation and iteration are key to success. When a model underperforms—whether it's a predictive model for customer segmentation or a campaign attribution model—marketers should test different approaches and experiment with new techniques, rather than over-tuning a single model. This iterative approach is at the core of effective marketing data science.
Marketing data is inherently complex, with numerous variables influencing outcomes. If one analysis or campaign isn't delivering results, don’t get bogged down trying to tweak every little detail. Explore different tactics. Experiment with new variables. Adjust your target audience, test different content formats, or try new channels. The key is to test, learn, and iterate quickly.
Why this matters: Marketing is not a one-size-fits-all approach, and different strategies will yield different results. Experimenting with multiple approaches helps identify what works best for your brand. By continuously testing and iterating, you can make data-driven adjustments that improve performance without getting stuck in a single approach that may not be effective.
Example: If your current customer segmentation isn’t yielding the desired engagement, don’t spend endless hours refining it. Instead, create a new segmentation model using different criteria, like customer behaviors or purchase frequency, and see if that drives better results.
Elevate Your Marketing Data Science Strategy with Machine Learning
Machine learning plays a pivotal role in advancing marketing data science, allowing agencies to process massive datasets, predict outcomes, and fine-tune campaign performance in real time. By leveraging machine learning models, you can go beyond surface-level insights—identifying patterns, predicting customer behavior, and automating optimizations, such as adjusting ad spend or refining audience targeting based on live data.
Practical applications of machine learning in marketing include automating report generation, where AI-driven assistants extract and analyze performance metrics without manual effort. Machine learning models can also assist in identifying key trends across campaigns or spotting potential issues, like declining engagement, early enough to course-correct before they impact results. Additionally, AI tools can help uncover insights hidden in complex data sets, from optimizing cross-channel performance to forecasting customer lifetime value or churn rates.
Why this matters: Machine learning reduces the time spent on repetitive tasks like data extraction and reporting, while providing deeper, more accurate insights. Agency product builders can leverage machine learning to develop smarter analytics platforms, allowing their teams to predict campaign performance or automate optimizations. AI helps ensure that marketing strategies are both data-driven and responsive, enabling you to quickly identify what’s working and make adjustments as needed.
Example: Suppose you're managing a multi-channel marketing campaign across paid search, social media, and display ads. By applying machine learning in media mix modeling, you can analyze the historical performance of each channel to predict which combination of media will deliver the highest return on investment. Machine learning models can identify how changes in ad spend across different platforms will impact overall conversions, allowing you to allocate your budget more effectively. This data-driven approach helps optimize your media mix in real time, ensuring that every dollar is working toward maximum campaign performance.
By applying these marketing data science principles—such as starting with simple models, conducting thorough EDA, trusting data-driven insights, and continuously experimenting—you can transform your marketing data into a strategic asset for driving growth and long-term success.